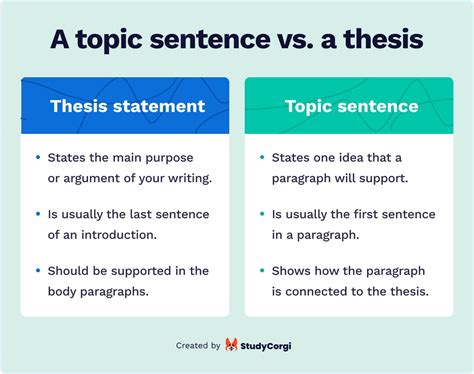
In statistics, width is a crucial idea that describes the unfold or variability of an information set. It measures the vary of values inside an information set, offering insights into the dispersion of the info factors. Calculating width is important for understanding the distribution and traits of an information set, enabling researchers and analysts to attract significant conclusions.
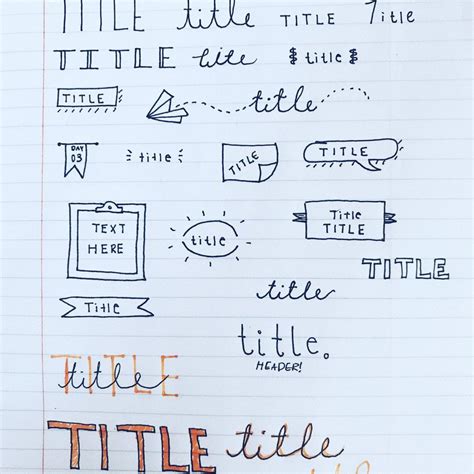
There are a number of methods to calculate width, relying on the particular kind of knowledge being analyzed. For a easy information set, the vary is a typical measure of width. The vary is calculated because the distinction between the utmost and minimal values within the information set. It offers a simple indication of the general unfold of the info however might be delicate to outliers.
For extra advanced information units, measures such because the interquartile vary (IQR) or commonplace deviation are extra applicable. The IQR is calculated because the distinction between the higher quartile (Q3) and the decrease quartile (Q1), representing the vary of values inside which the center 50% of the info falls. The usual deviation is a extra complete measure of width, bearing in mind the distribution of all information factors and offering a statistical estimate of the typical deviation from the imply. The selection of width measure is determined by the particular analysis query and the character of the info being analyzed.
Introduction to Width in Statistics
In statistics, width refers back to the vary of values {that a} set of knowledge can take. It’s a measure of the unfold or dispersion of knowledge, and it may be used to check the variability of various information units. There are a number of other ways to measure width, together with:
- Vary: The vary is the only measure of width. It’s calculated by subtracting the minimal worth from the utmost worth within the information set.
- Interquartile vary (IQR): The IQR is the vary of the center 50% of the info. It’s calculated by subtracting the primary quartile (Q1) from the third quartile (Q3).
- Customary deviation: The usual deviation is a extra refined measure of width that takes into consideration the distribution of the info. It’s calculated by discovering the sq. root of the variance, which is the typical of the squared deviations from the imply.
The desk beneath summarizes the completely different measures of width and their formulation:
Measure of width |
System |
Vary |
Most worth – Minimal worth |
IQR |
Q3 – Q1 |
Customary deviation |
√Variance |
The selection of which measure of width to make use of is determined by the particular function of the evaluation. The vary is a straightforward and easy-to-understand measure, however it may be affected by outliers. The IQR is much less affected by outliers than the vary, however it isn’t as simple to interpret. The usual deviation is essentially the most complete measure of width, however it’s tougher to calculate than the vary or IQR.
Measuring the Dispersion of Knowledge
Dispersion refers back to the unfold or variability of knowledge. It measures how a lot the info values differ from the central tendency, offering insights into the consistency or variety inside a dataset.
Vary
The vary is the only measure of dispersion. It’s calculated by subtracting the minimal worth from the utmost worth within the dataset. The vary offers a fast and straightforward indication of the info’s unfold, however it may be delicate to outliers, that are excessive values that considerably differ from the remainder of the info.
Interquartile Vary (IQR)
The interquartile vary (IQR) is a extra strong measure of dispersion than the vary. It’s calculated by discovering the distinction between the third quartile (Q3) and the primary quartile (Q1). The IQR represents the center 50% of the info and is much less affected by outliers. It offers a greater sense of the everyday unfold of the info than the vary.
Calculating the IQR
To calculate the IQR, comply with these steps:
- Organize the info in ascending order.
- Discover the median (Q2), which is the center worth of the dataset.
- Discover the median of the values beneath the median (Q1).
- Discover the median of the values above the median (Q3).
- Calculate the IQR as IQR = Q3 – Q1.
Three Widespread Width Measures
In statistics, there are three generally used measures of width. These are the vary, the interquartile vary, and the usual deviation. The vary is the distinction between the utmost and minimal values in an information set. The interquartile vary (IQR) is the distinction between the third quartile (Q3) and the primary quartile (Q1) of an information set. The commonplace deviation (σ) is a measure of the variability or dispersion of an information set. It’s calculated by discovering the sq. root of the variance, which is the typical of the squared variations between every information level and the imply.
Vary
The vary is the only measure of width. It’s calculated by subtracting the minimal worth from the utmost worth in an information set. The vary might be deceptive if the info set incorporates outliers, as these can inflate the vary. For instance, if we’ve an information set of {1, 2, 3, 4, 5, 100}, the vary is 99. Nonetheless, if we take away the outlier (100), the vary is just 4.
Interquartile Vary
The interquartile vary (IQR) is a extra strong measure of width than the vary. It’s much less affected by outliers and is an efficient measure of the unfold of the central 50% of the info. The IQR is calculated by discovering the distinction between the third quartile (Q3) and the primary quartile (Q1) of an information set. For instance, if we’ve an information set of {1, 2, 3, 4, 5, 6, 7, 8, 9, 10}, the median is 5, Q1 is 3, and Q3 is 7. The IQR is subsequently 7 – 3 = 4.
Customary Deviation
The usual deviation (σ) is a measure of the variability or dispersion of an information set. It’s calculated by discovering the sq. root of the variance, which is the typical of the squared variations between every information level and the imply. The usual deviation can be utilized to check the variability of various information units. For instance, if we’ve two information units with the identical imply however completely different commonplace deviations, the info set with the bigger commonplace deviation has extra variability.
Calculating Vary
The vary is a straightforward measure of variability calculated by subtracting the smallest worth in a dataset from the biggest worth. It provides an total sense of how unfold out the info is, however it may be affected by outliers (excessive values). To calculate the vary, comply with these steps:
- Put the info in ascending order.
- Subtract the smallest worth from the biggest worth.
For instance, you probably have the next information set: 5, 10, 15, 20, 25, 30, the vary is 30 – 5 = 25.
Calculating Interquartile Vary
The interquartile vary (IQR) is a extra strong measure of variability that’s much less affected by outliers than the vary. It’s calculated by subtracting the worth of the primary quartile (Q1) from the worth of the third quartile (Q3). To calculate the IQR, comply with these steps:
- Put the info in ascending order.
- Discover the median (the center worth). If there are two center values, calculate the typical of the 2.
- Divide the info into two halves: the decrease half and the higher half.
- Discover the median of the decrease half (Q1).
- Discover the median of the higher half (Q3).
- Subtract Q1 from Q3.
For instance, you probably have the next information set: 5, 10, 15, 20, 25, 30, the median is 17.5. The decrease half of the info set is: 5, 10, 15. The median of the decrease half is Q1 = 10. The higher half of the info set is: 20, 25, 30. The median of the higher half is Q3 = 25. Due to this fact, the IQR is Q3 – Q1 = 25 – 10 = 15.
Measure of Variability |
System |
Interpretation |
Vary |
Most worth – Minimal worth |
Total unfold of the info, however affected by outliers |
Interquartile Vary (IQR) |
Q3 – Q1 |
Unfold of the center 50% of the info, much less affected by outliers |
Calculating Variance
Variance is a measure of how unfold out a set of knowledge is. It’s calculated by discovering the typical of the squared variations between every information level and the imply. The variance is then the sq. root of this common.
Calculating Customary Deviation
Customary deviation is a measure of how a lot a set of knowledge is unfold out. It’s calculated by taking the sq. root of the variance. The usual deviation is expressed in the identical items as the unique information.
Deciphering Variance and Customary Deviation
The variance and commonplace deviation can be utilized to know how unfold out a set of knowledge is. A excessive variance and commonplace deviation point out that the info is unfold out over a variety of values. A low variance and commonplace deviation point out that the info is clustered near the imply.
Statistic |
System |
Variance |
s2 = Σ(x – μ)2 / (n – 1) |
Customary Deviation |
s = √s2 |
Instance: Calculating Variance and Customary Deviation
Contemplate the next set of knowledge: 10, 12, 14, 16, 18, 20.
The imply of this information set is 14.
The variance of this information set is:
“`
s2 = (10 – 14)2 + (12 – 14)2 + (14 – 14)2 + (16 – 14)2 + (18 – 14)2 + (20 – 14)2 / (6 – 1) = 10.67
“`
The usual deviation of this information set is:
“`
s = √10.67 = 3.26
“`
This means that the info is unfold out over a spread of three.26 items from the imply.
Selecting the Acceptable Width Measure
1. Vary
The vary is the only width measure, and it’s calculated by subtracting the minimal worth from the utmost worth. The vary is simple to calculate, however it may be deceptive if there are outliers within the information. Outliers are excessive values which are a lot bigger or smaller than the remainder of the info. If there are outliers within the information, the vary can be inflated and it’ll not be an excellent measure of the everyday width of the info.
2. Interquartile Vary (IQR)
The IQR is a extra strong measure of width than the vary. The IQR is calculated by subtracting the decrease quartile from the higher quartile. The decrease quartile is the median of the decrease half of the info, and the higher quartile is the median of the higher half of the info. The IQR isn’t affected by outliers, and it’s a higher measure of the everyday width of the info than the vary.
3. Customary Deviation
The usual deviation is a measure of how a lot the info is unfold out. The usual deviation is calculated by taking the sq. root of the variance. The variance is the typical of the squared variations between every information level and the imply. The usual deviation is an efficient measure of the everyday width of the info, however it may be affected by outliers.
4. Imply Absolute Deviation (MAD)
The MAD is a measure of how a lot the info is unfold out. The MAD is calculated by taking the typical of absolutely the variations between every information level and the median. The MAD isn’t affected by outliers, and it’s a good measure of the everyday width of the info.
5. Coefficient of Variation (CV)
The CV is a measure of how a lot the info is unfold out relative to the imply. The CV is calculated by dividing the usual deviation by the imply. The CV is an efficient measure of the everyday width of the info, and it isn’t affected by outliers.
6. Percentile Vary
The percentile vary is a measure of the width of the info that’s based mostly on percentiles. The percentile vary is calculated by subtracting the decrease percentile from the higher percentile. The percentile vary is an efficient measure of the everyday width of the info, and it isn’t affected by outliers. Probably the most generally used percentile vary is the 95% percentile vary, which is calculated by subtracting the fifth percentile from the ninety fifth percentile. This vary measures the width of the center 90% of the info.
Width Measure |
System |
Robustness to Outliers |
Vary |
Most – Minimal |
Not strong |
IQR |
Higher Quartile – Decrease Quartile |
Sturdy |
Customary Deviation |
√(Variance) |
Not strong |
MAD |
Common of Absolute Variations from Median |
Sturdy |
CV |
Customary Deviation / Imply |
Not strong |
Percentile Vary (95%) |
ninety fifth Percentile – fifth Percentile |
Sturdy |
Purposes of Width in Statistical Evaluation
Knowledge Summarization
The width of a distribution offers a concise measure of its unfold. It helps determine outliers and evaluate the variability of various datasets, aiding in information exploration and summarization.
Confidence Intervals
The width of a confidence interval displays the precision of an estimate. A narrower interval signifies a extra exact estimate, whereas a wider interval suggests better uncertainty.
Speculation Testing
The width of a distribution can affect the outcomes of speculation assessments. A wider distribution reduces the ability of the check, making it much less prone to detect important variations between teams.
Quantile Calculation
The width of a distribution determines the gap between quantiles (e.g., quartiles). By calculating quantiles, researchers can determine values that divide the info into equal proportions.
Outlier Detection
Values that lie far outdoors the width of a distribution are thought of potential outliers. Figuring out outliers helps researchers confirm information integrity and account for excessive observations.
Mannequin Choice
The width of a distribution can be utilized to check completely different statistical fashions. A mannequin that produces a distribution with a narrower width could also be thought of a greater match for the info.
Chance Estimation
The width of a distribution impacts the likelihood of a given worth occurring. A wider distribution spreads likelihood over a bigger vary, leading to decrease chances for particular values.
Deciphering Width in Actual-World Contexts
Calculating width in statistics offers worthwhile insights into the distribution of knowledge. Understanding the idea of width permits researchers and analysts to attract significant conclusions and make knowledgeable selections based mostly on information evaluation.
Listed here are some widespread functions the place width performs a vital function in real-world contexts:
Inhabitants Surveys
In inhabitants surveys, width can point out the unfold or vary of responses inside a inhabitants. A wider distribution suggests better variability or variety within the responses, whereas a narrower distribution implies a extra homogenous inhabitants.
Market Analysis
In market analysis, width can assist decide the audience and the effectiveness of promoting campaigns. A wider distribution of buyer preferences or demographics signifies a various audience, whereas a narrower distribution suggests a extra particular buyer base.
High quality Management
In high quality management, width is used to observe product or course of consistency. A narrower width usually signifies higher consistency, whereas a wider width could point out variations or defects within the course of.
Predictive Analytics
In predictive analytics, width might be essential for assessing the accuracy and reliability of fashions. A narrower width suggests a extra exact and dependable mannequin, whereas a wider width could point out a much less correct or much less secure mannequin.
Monetary Evaluation
In monetary evaluation, width can assist consider the chance and volatility of monetary devices or investments. A wider distribution of returns or costs signifies better danger, whereas a narrower distribution implies decrease danger.
Medical Analysis
In medical analysis, width can be utilized to check the distribution of well being outcomes or affected person traits between completely different teams or therapies. Wider distributions could recommend better heterogeneity or variability, whereas narrower distributions point out better similarity or homogeneity.
Instructional Evaluation
In instructional evaluation, width can point out the vary or unfold of pupil efficiency on exams or assessments. A wider distribution implies better variation in pupil skills or efficiency, whereas a narrower distribution suggests a extra homogenous pupil inhabitants.
Environmental Monitoring
In environmental monitoring, width can be utilized to evaluate the variability or change in environmental parameters, corresponding to air air pollution or water high quality. A wider distribution could point out better variability or fluctuations within the setting, whereas a narrower distribution suggests extra secure or constant situations.
Limitations of Width Measures
Width measures have sure limitations that needs to be thought of when decoding their outcomes.
1. Sensitivity to Outliers
Width measures might be delicate to outliers, that are excessive values that don’t signify the everyday vary of the info. Outliers can inflate the width, making it seem bigger than it truly is.
2. Dependence on Pattern Measurement
Width measures are depending on the pattern measurement. Smaller samples have a tendency to supply wider ranges, whereas bigger samples sometimes have narrower ranges. This makes it troublesome to check width measures throughout completely different pattern sizes.
3. Affect of Distribution Form
Width measures are additionally influenced by the form of the distribution. Distributions with numerous outliers or a protracted tail are likely to have wider ranges than distributions with a extra central peak and fewer outliers.
4. Alternative of Measure
The selection of width measure can have an effect on the outcomes. Totally different measures present completely different interpretations of the vary of the info, so it is very important choose the measure that finest aligns with the analysis query.
5. Multimodality
Width measures might be deceptive for multimodal distributions, which have a number of peaks. In such instances, the width could not precisely signify the unfold of the info.
6. Non-Regular Distributions
Width measures are sometimes designed for regular distributions. When the info is non-normal, the width is probably not a significant illustration of the vary.
7. Skewness
Skewed distributions can produce deceptive width measures. The width could underrepresent the vary for skewed distributions, particularly if the skewness is excessive.
8. Models of Measurement
The items of measurement used for the width measure needs to be thought of. Totally different items can result in completely different interpretations of the width.
9. Contextual Issues
When decoding width measures, it is very important think about the context of the analysis query. The width could have completely different meanings relying on the particular analysis objectives and the character of the info. It’s important to fastidiously consider the restrictions of the width measure within the context of the research.
Superior Strategies for Calculating Width
Calculating width in statistics is a elementary idea used to measure the variability or unfold of a distribution. Right here we discover some superior methods for calculating width:
Vary
The vary is the distinction between the utmost and minimal values in a dataset. Whereas intuitive, it may be affected by outliers, making it much less dependable for skewed distributions.
Interquartile Vary (IQR)
The IQR is the distinction between the higher and decrease quartiles (Q3 and Q1). It offers a extra strong measure of width, much less inclined to outliers than the vary.
Customary Deviation
The usual deviation is a generally used measure of unfold. It considers the deviation of every information level from the imply. A bigger commonplace deviation signifies better variability.
Variance
Variance is the squared worth of the usual deviation. It offers an alternate measure of unfold on a distinct scale.
Coefficient of Variation (CV)
The CV is a standardized measure of width. It’s the usual deviation divided by the imply. The CV permits for comparisons between datasets with completely different items.
Percentile Vary
The percentile vary is the distinction between the p-th and (100-p)-th percentiles. By selecting completely different values of p, we receive numerous measures of width.
Imply Absolute Deviation (MAD)
The MAD is the typical of absolutely the deviations of every information level from the median. It’s much less affected by outliers than commonplace deviation.
Skewness
Skewness is a measure of the asymmetry of a distribution. A optimistic skewness signifies a distribution with an extended proper tail, whereas a destructive skewness signifies an extended left tail. Skewness can affect the width of a distribution.
Kurtosis
Kurtosis is a measure of the flatness or peakedness of a distribution. A optimistic kurtosis signifies a distribution with a excessive peak and heavy tails, whereas a destructive kurtosis signifies a flatter distribution. Kurtosis may have an effect on the width of a distribution.
Approach |
System |
Description |
Vary |
Most – Minimal |
Distinction between the biggest and smallest values. |
Interquartile Vary (IQR) |
Q3 – Q1 |
Distinction between the higher and decrease quartiles. |
Customary Deviation |
√(Σ(x – μ)² / (n-1)) |
Sq. root of the typical squared variations from the imply. |
Variance |
Σ(x – μ)² / (n-1) |
Squared commonplace deviation. |
Coefficient of Variation (CV) |
Customary Deviation / Imply |
Standardized measure of unfold. |
Percentile Vary |
P-th Percentile – (100-p)-th Percentile |
Distinction between specified percentiles. |
Imply Absolute Deviation (MAD) |
Σ|x – Median| / n |
Common absolute distinction from the median. |
Skewness |
(Imply – Median) / Customary Deviation |
Measure of asymmetry of distribution. |
Kurtosis |
(Σ(x – μ)⁴ / (n-1)) / Customary Deviation⁴ |
Measure of flatness or peakedness of distribution. |
How To Calculate Width In Statistics
In statistics, the width of a category interval is the distinction between the higher and decrease class limits. It’s used to group information into intervals, which makes it simpler to research and summarize the info. To calculate the width of a category interval, subtract the decrease class restrict from the higher class restrict.
For instance, if the decrease class restrict is 10 and the higher class restrict is 20, the width of the category interval is 10.
Folks Additionally Ask About How To Calculate Width In Statistics
What’s a category interval?
A category interval is a spread of values which are grouped collectively. For instance, the category interval 10-20 consists of all values from 10 to twenty.
How do I select the width of a category interval?
The width of a category interval needs to be giant sufficient to incorporate a big variety of information factors, however sufficiently small to offer significant data. rule of thumb is to decide on a width that’s about 10% of the vary of the info.
What’s the distinction between a category interval and a frequency distribution?
A category interval is a spread of values, whereas a frequency distribution is a desk that exhibits the variety of information factors that fall into every class interval.